Data Related Technologies: How Not to Get Confused
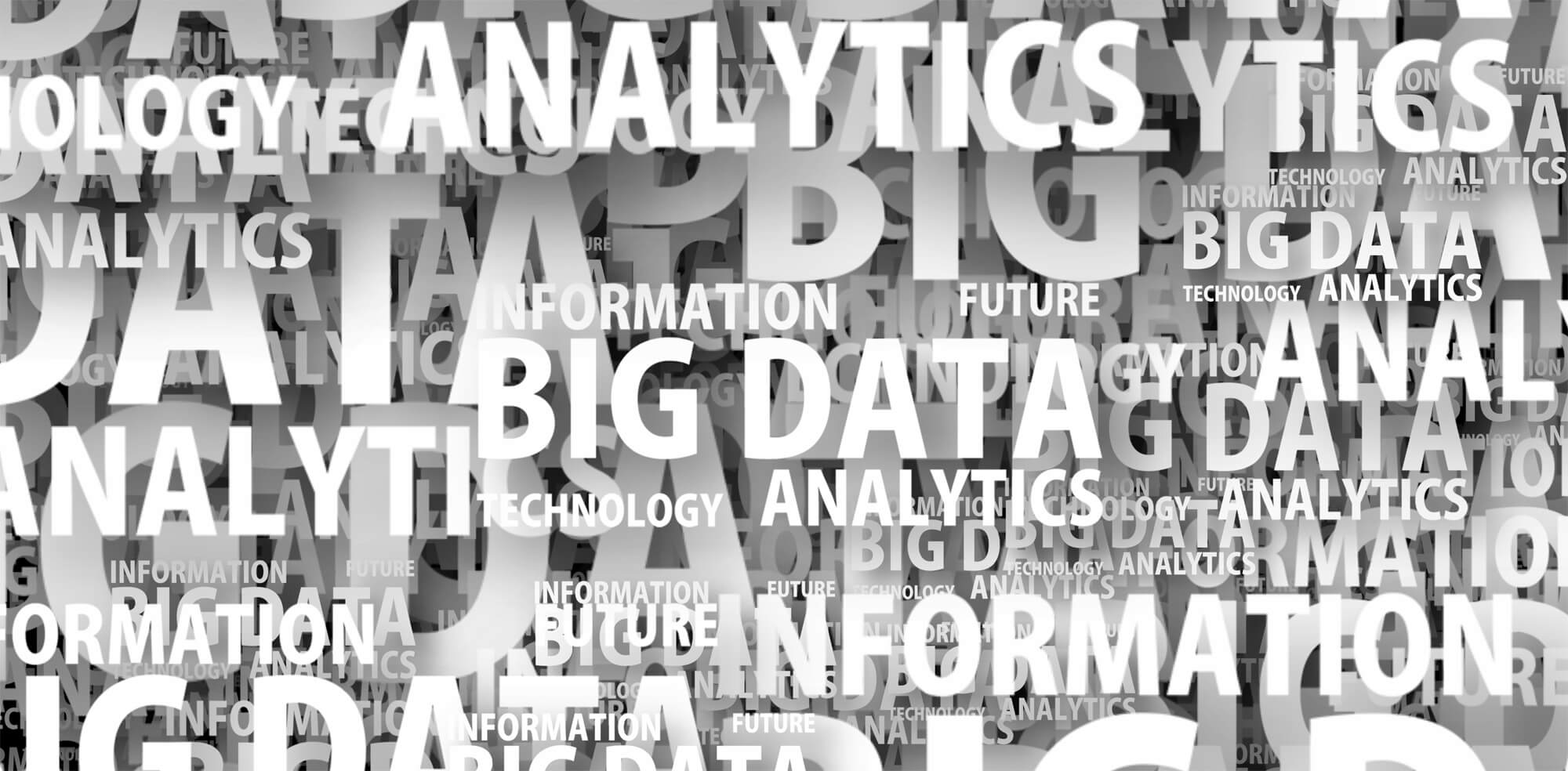
Data has always served as a basis for business decisions in all industries across the globe. With new digital data sources in the modern world, the volume of data grows exponentially every year and it’s becoming far beyond the abilities of a human to effectively process and analyze it.
In order to work with this data, better understand it and get the most out of it, a number of brand-new fields and technologies have introduced that focus on data dissection and further analysis.
In this article, we will talk about Big Data, Data Analytics, and Data Science. It’s easy to get confused by all the relatively new terms so we will have a close look at each and will try to answer a question: is there actually a difference between Data Science, Data Analytics, and Big Data.
Big Data
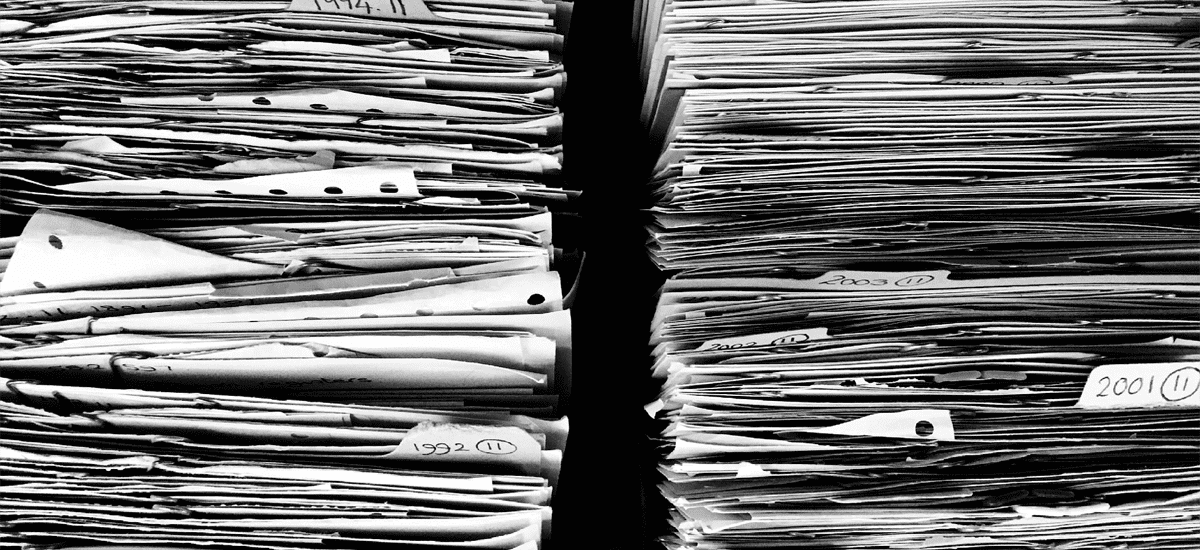
Big Data is about managing and processing huge volumes of data. It encompasses all the data that is available:
- unstructured (i.e. data from social media);
- semi-structured (i.e. XML files);
- structured.
So basically, Big Data is Big Data sets and specific software to process them. And there are three extremely important qualities of Big Data:
- volume: the actual amount of data;
- velocity: the rate at which the data is received;
- variety: meaning, there are many different data types.
One can actually say that Big Data is also a subset of Data Science that is comprised of the methods on data collection, systematization, and proper storage in order to easily process this data in the future. It’s often used as a synonymous of Data Science, though in fact, Data Science is a wider field of study.
The most important value of Big Data is that it can actually produce new data, which is potentially more valuable.
Big Data brings great value to many industries. One of the most popular examples of Big Data usage is processing the customers’ data and purchase history in order to generate new data: e.g. customer behavior statistics, learn from it and adjust the marketing strategy correspondingly.
Data Analytics
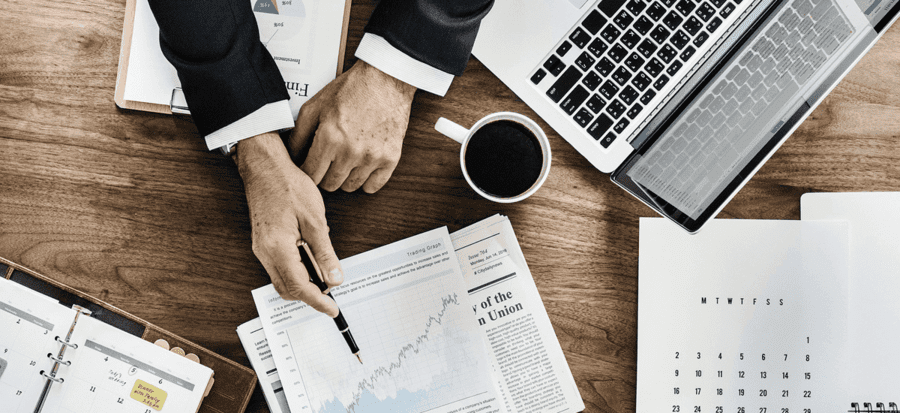
Data Analytics is a comprehensive field of study that involves many processes: data cleansing and its further transformation in order to use the analyzed data for one’s benefit.
There are four types of Data Analytics:
- descriptive: helps businesses understand what past events led to the present status;
- diagnostic: answers why something happened;
- predictive: can draw forecasts on what is likely going to happen in the future, based on the historical data;
- prescriptive: provides the best solution for the future.
Modern businesses widely use Data Analytics because it helps them make accurate decisions regarding future growth and other important aspects of development. With the help of Data Analytics tools, businesses can:
- find out what went wrong in the past and how to avoid such mistakes in the future;
- better understand the customers and provide them with more relevant services;
- point out the areas of growth possibilities;
- make forecasts and determine risks;
- examine the health of entire systems;
- detect dependencies.
Data Analytics is a great tool for those businesses that don’t want to do any guesswork and instead, focus on precise resolution of any issues.
Data Science
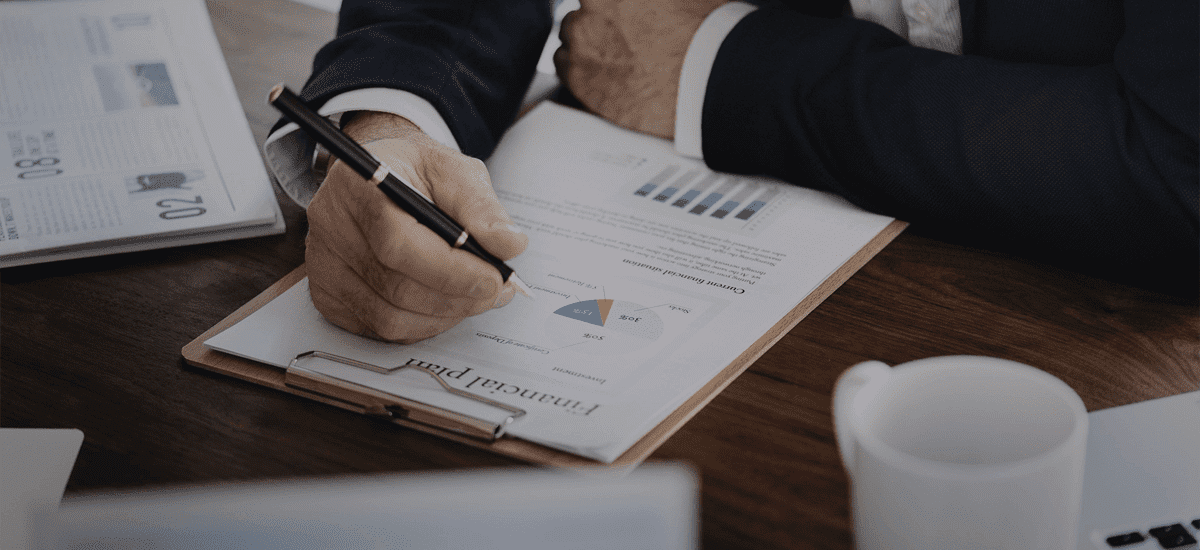
Finally, we’ve come to Data Science.
Data Science is a field that comprises everything data-related: methods, algorithms, processes. Data Science is aimed at a deep understanding of the data in order to draw valuable insights from it.
Because Data Science covers all aspects of work with the data, it includes the following processes:
- data collection;
- data distribution;
- data processing;
- data visualization;
- deployment of data into production.
Because Data Science is a whole field of study, businesses rarely use all the methods and processes that it has at once. Instead, a company formulates its goals and, based on them, chooses the best way to resolve the issue.
Professionals who work in this field are called data scientists. It is a data scientist’s job to preliminary analyze the collected data in order to assume the value that can be extracted out of it and suggest a processing model that will provide the desired results.
Overall, Data Science is an incredibly vast field and comprises many techniques and subsets, including trending machine learning and data mining, computer vision and natural language processing.
So What is the Relationship Between the Three?
One could say that Data Analytics is basically a use case of Big Data, which, in its turn, is a subset of Data Science. Yet Big Data term is often used as a synonym for Data Science which is also not wrong.
Still didn’t get the difference? It’s fun to say, but the truth is that the difference is only in the context of meaning. In the end, it’s all about the same: getting value from the data.
Final Word
Correct usage of data can bring incredible results. Automation of processes, personalized assistance, predictive analytics, and data-driven decision making – these are just a few examples of how data impacts our everyday life.
Whether you want to boost the performance of business processes or look for new ways to contribute to a specific industry, data is the key.