Can Machine Learning Really Estimate How Well You Know a Foreign Language?
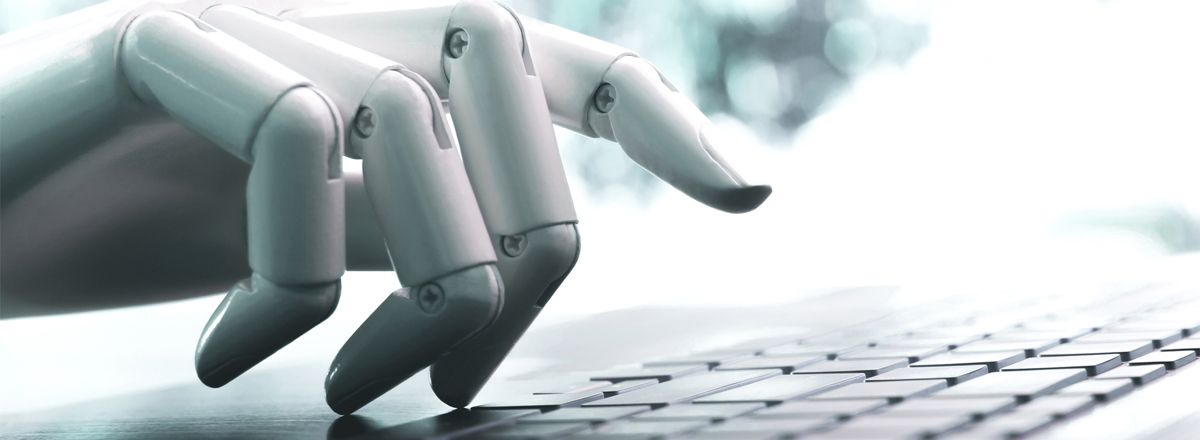
It is known that Machine Learning allows to analyze Big Data and predict behavior or probability of specific events almost certainly, but it is all about the future. So, the question is whether it is possible for ML to assess the current situation in real-time?
Possibility to track progress is a key inducement to better outcomes. For instance, a marathon racer focuses on endurance enhancing to be able to overcome large distances. Due to progress level tracking, we can set clear and measurable goals and move towards the final destination, dividing the road into comprehensible stages.
But we come across some difficulties when dealing with knowledge acquisition processes, like learning a new language. Impossibility of accurate measure of the knowledge gained and defining of the level reached complexifies tracking of progress, because of the learning processes individuality. It becomes even more complicated when a person has a certain aim, like the ability to maintain a conversation with people at conferences.
Due to the inability to maintain accurate progress tracking and nonexistence of the final destination, people get demotivated and likely to abandon the language learning process. It is easy to evaluate the number of words learned, but it becomes challenging to estimate the level of grammar acquired or communication skills developed.
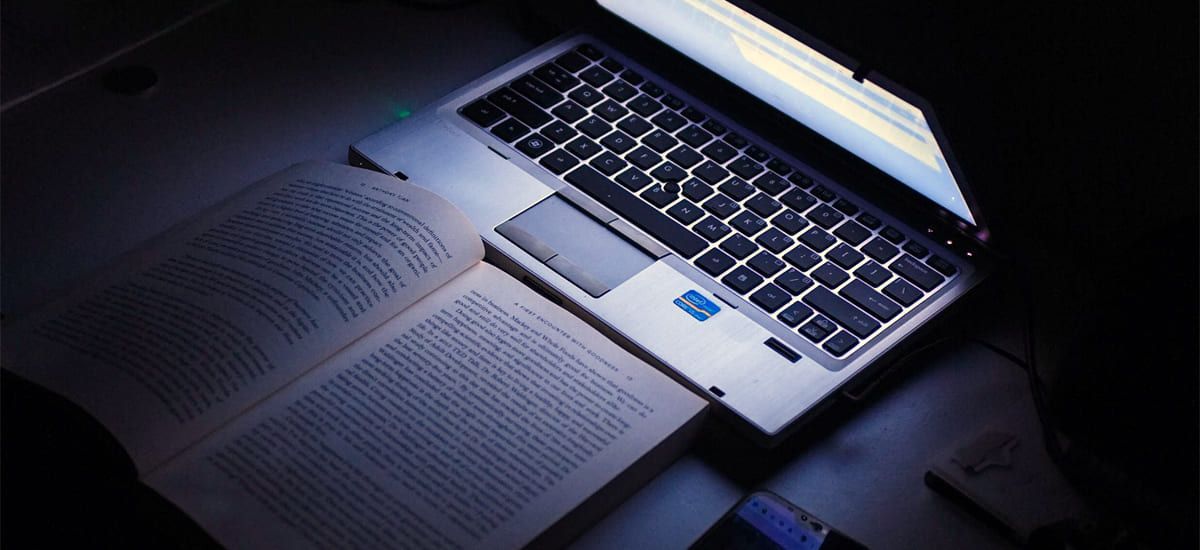
Skills fragmentation
How much do you remember from what you learned at school? How much of it do you use in your everyday life? It’s pretty obvious that we don’t use most of what we learned. The reason is that there is no need for that.
The same applies to learn languages. As it was already stated, we learn a new language to resolve a specific problem. But it will depend on the problem. Different problems require different skills. You can’t learn all the skills equally well, some of them will prevail above others, depending on your needs.
Furthermore, language is not as precise as Math. If you learn a few tenses, you will not become an expert in grammar but at the same time, it doesn’t mean that you don’t know anything at all. In conclusion, we can’t evaluate language learning skills with the linear method.
There was a massive study which was conducted by the Pearson company within its Global Scale of English research. More than 6000 teachers took part in that survey. They had to identify the basic English language knowledge and skills and to range it from the easiest to the most difficult one to learn. This very study became a foundation for the ML model that was developed by a Skyeng company.
When studying a new language, it’s important to learn grammar, learn how to read and write, have listening comprehension, and develop your oral skills.
Each of these main components includes a range of skills required to learn the full component. To completely understand a language, one must have a real-life application of those skills. Like, for example, understanding a conversation or asking for directions.
The Skyeng ML model includes over 1200 of such unique skills, selected for each student depending on their objective and then adjusting in the process of learning.
Now just think how much time and resources one needs to master 100 skills. That’s where Machine Learning steps in, setting dependencies between the skills. Those may be:
- Grammar: if you can ask questions in the present, he will ask those correctly in the future;
- Cognitive: if you can speak, you may understand it from another person’s speech;
- Specific: one skill is related to another (e. if you can call a cab, you can book a plane ticket).
The model is meant to show a clear view of a student’s knowledge on the basis of skills that are analyzed in a repetitive manner and evaluated with the help of relevant skills. Thus, just a part of skills is enough to get information about one’s knowledge. Various interactions with the students are also included in the operation of this model. Just as a student commits an action within a platform, he will at once get feedback on progress. In this way, the model collects all the information, combines it with the skills from the database and so evaluates one’s progress.
But this model also has one and only disadvantage. It’s hard enough to evaluate a student’s knowledge about grammar and writing when the model mode is automatic. For instance, if a student pronounces some words incorrectly due to his accent, the model can confuse them with words that sound similar instead of finding a mistake. A very huge amount of time can be needed to develop such a model. But for this time, it operates in a semi-automatic mode in collaboration with educators who give rankings.
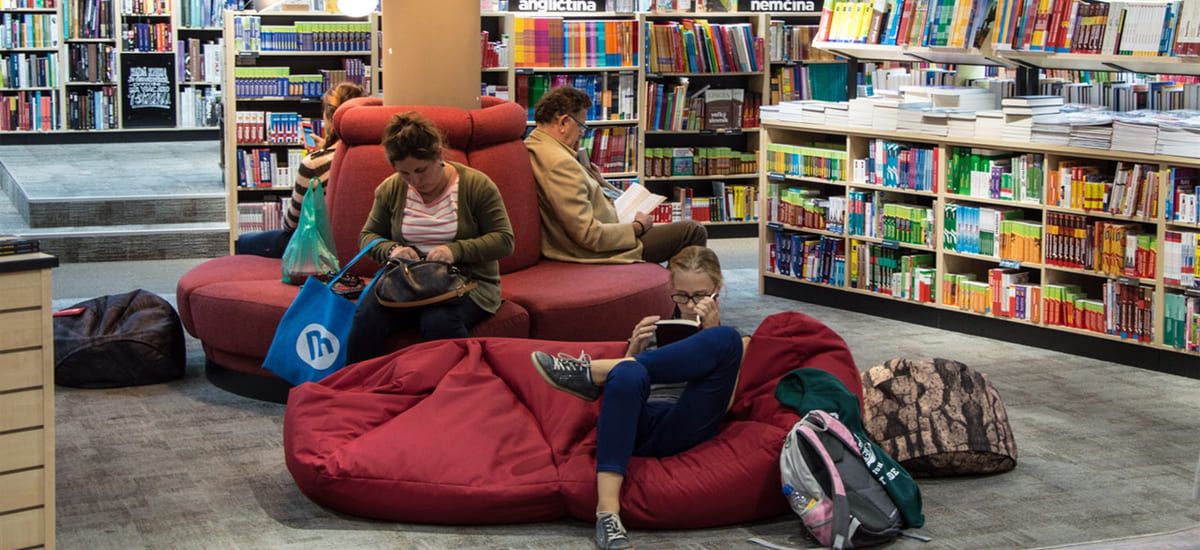
More insights into the educational process
Every newcomer student puts learning as the first priority task. Our designers aware of it, therefore they thoroughly control the distribution of teachers and courses by the model for suitability with user’s needs. Moreover, the skills assessment model allows teachers to suggest their students a corresponding set of skills to work on.
Starting from the very first lesson users are provided with useful information as the model can evaluate a student's abilities and measure the gap between the skills which are already acquired and with the desired ones. The model is in constant upgrade mode to provide better assistance for students. Currently, it’s in a beta phase, however automated work and active adjustment to user’s necessities are the main targets of the final version.